Why machine vision is the next big thing in manufacturing
Everyone’s heard the saying, "there’s more than meets the eye," and in manufacturing, vision technology certainly proves this to be true. While machine vision uptake is expected to increase significantly over the coming years, implementation challenges are holding back manufacturers from tapping into its game-changing potential.
Machine vision technology, or industrial machine vision, combines hardware and software to capture and interpret visual information through cameras and sophisticated processing algorithms, enabling intelligent decision-making.
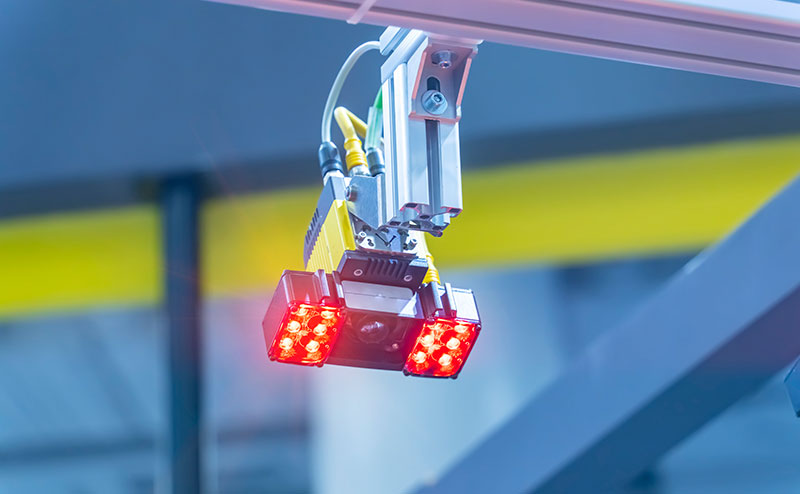
It’s currently used in industrial settings for quality control, defect detection, product sorting, tracking, positioning, measurement, and barcode reading.
Its usage is projected to increase by 37 per cent over the next two years, with survey respondents reporting expected growth from the current 46 per cent to an anticipated 63 per cent of potential applications in the near future.
Although it offers significant opportunities to boost productivity and efficiency in manufacturing, its implementation can be challenging, especially for SMEs.
Terminology inconsistencies
A notable inconsistency is in the terminology used to describe machine vision across different countries and regions.
Research indicates that while the term "machine vision" is widely recognised in countries like the USA, India, and Germany, it is far less common in France, where only eight per cent of companies use this term. Instead, the term "vision sensors" is more prevalent.
Globally, the most frequently used terms include "machine vision" and "vision sensors," but other phrases such as "vision systems," "industrial sensors," "computer vision," "visual inspection," and "smart cameras" are also in circulation.
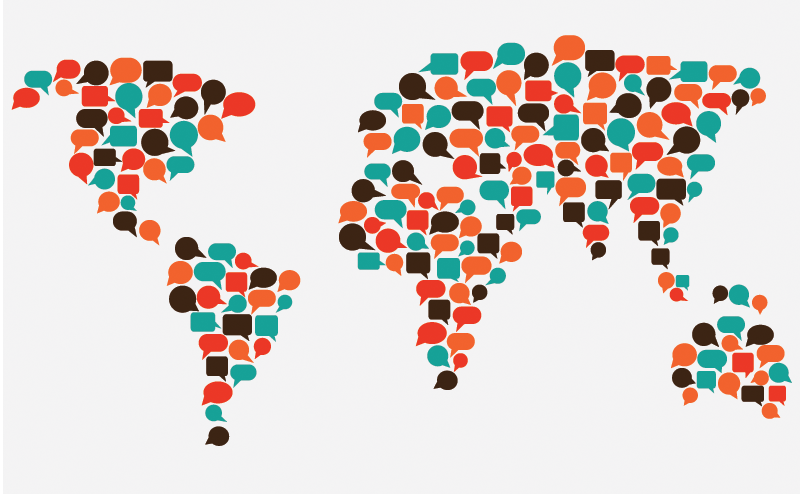
This lack of standard terminology complicates the market in several ways, adding complexity to the buying process for companies, many of which already find the decision-making process challenging.
Additionally, the inconsistent language creates barriers to global discussions and collaboration in the field. Establishing a consensus on naming and terminology can simplify these processes and facilitate clearer communication across industries and borders.
High cost
Aside from terminology, there are also high initial costs for adopting advanced technologies like machine vision and related fields, such as machine learning (ML) and artificial intelligence (AI) in manufacturing.
A basic machine vision setup, designed solely to identify defects or confirm if a product meets certain criteria, is relatively straightforward and less costly. This setup acts as a highly capable "inspector," flagging whether parts pass or fail based on predefined visual parameters.
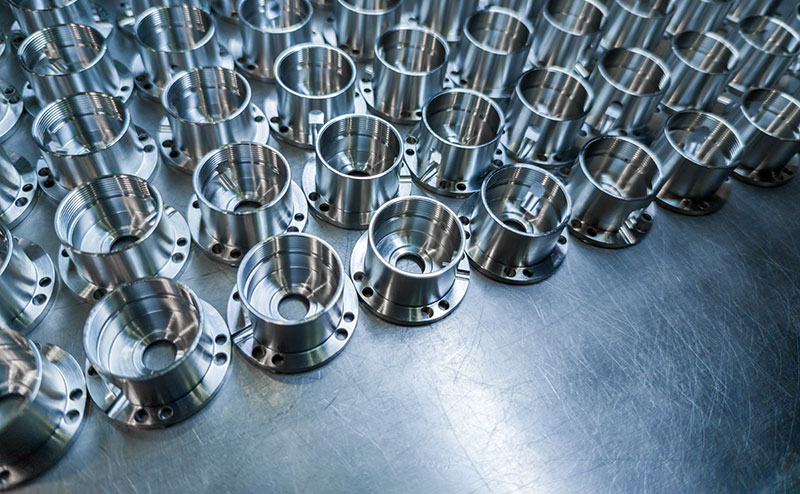
However, incorporating ML introduces additional capabilities and complexities. ML systems learn from the data collected by the vision system to identify patterns, distinguish between normal textures and defects, and even infer possible root causes of recurring issues.
This transition from basic machine vision to a more intelligent ML-driven approach comes with higher costs but also significant advantages.
For example, deploying a simple ML model to work alongside a machine vision system has a starting cost of around $60,000 over the first five years. This would cover the foundational requirements, but such a bare-bones system may struggle with scalability and lack features to handle more advanced tasks or future growth.
On the other hand, opting for a more comprehensive, scalable framework capable of deploying multiple AI models involves a higher initial investment — approximately $95,000 over the same period. This setup not only supports the initial vision and ML model but also provides the infrastructure for additional models in the future.
With this approach, the cost of deploying subsequent models decreases significantly. The second model would cost around $24,000, the third about $14,000, and the incremental cost of adding future models continues to drop.
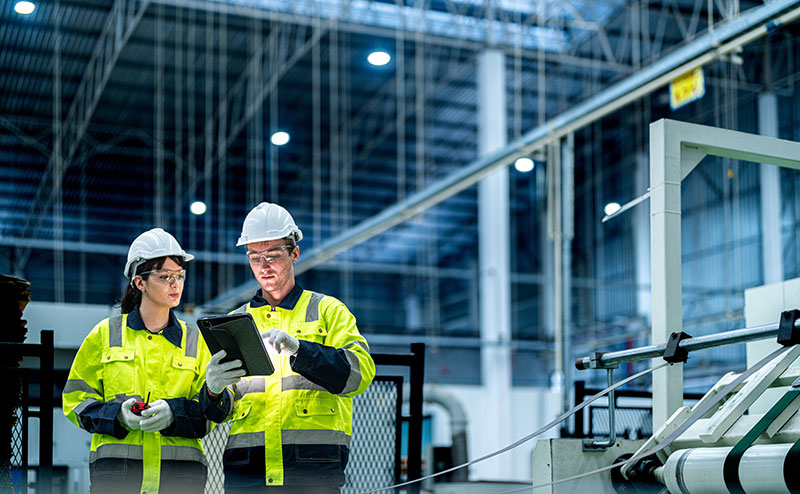
The key distinction here lies in the capabilities. Basic machine vision focuses on real-time visual assessments and decision-making, while ML enhances these systems by enabling them to learn, adapt, and provide deeper insights over time.
Though the upfront costs are higher for ML-integrated systems, the long-term benefits of flexibility, efficiency, and scalability make it an attractive option.
High reward
High cost often leads to high reward, which is certainly the case with machine vision. As factories turn to automation, machine vision is becoming the "eyes and ears" of machines, enabling real-time, accurate visual assessments of product quality, defect detection, and packaging.
For example, PackCheck has developed an innovative inspection platform for food producers with multiple production lines. Their machine-vision-based system offers flexibility and speed, allowing it to inspect various products quickly, even in cleanroom environments.
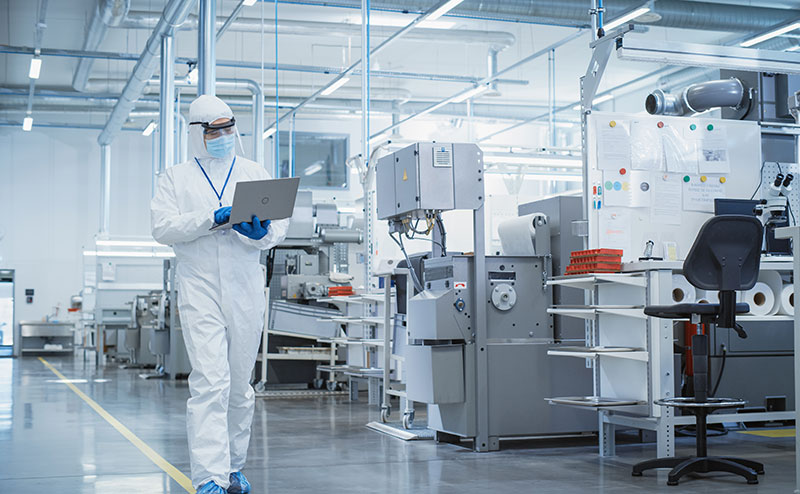
The main goal of companies using PackCheck's system is to minimise recall risks via inline quality control. By identifying recurring defects, the platform ensures thorough product inspection while providing valuable insights into the production process, helping companies to optimise operations, reduce waste, and prevent costly recalls.
Elsewhere, vision technology is enabling factories to transition to dark environments, also known as “lights out manufacturing,” which are designed to monitor and manage high-volume production with minimal human intervention.
With open standards integration, such as Beckhoff’s EtherCAT, vision technology can also connect seamlessly with other systems, enabling greater flexibility and scalability that help to tackle issues, such as the skills gap.
Overcoming barriers
As a result, there is an overwhelming consensus of 94 per cent among decision-makers that organisations would benefit from the potential applications of machine vision.
It’s also agreed among respondents that support will play a key role in the future, with 80 per cent saying their company needs guidance and assistance integrating machine vision with existing systems.
At Foxmere, we work closely with our partners to demystify machine vision and make its adoption accessible and effective for manufacturers of all sizes, from Fortune 500s to SMEs.
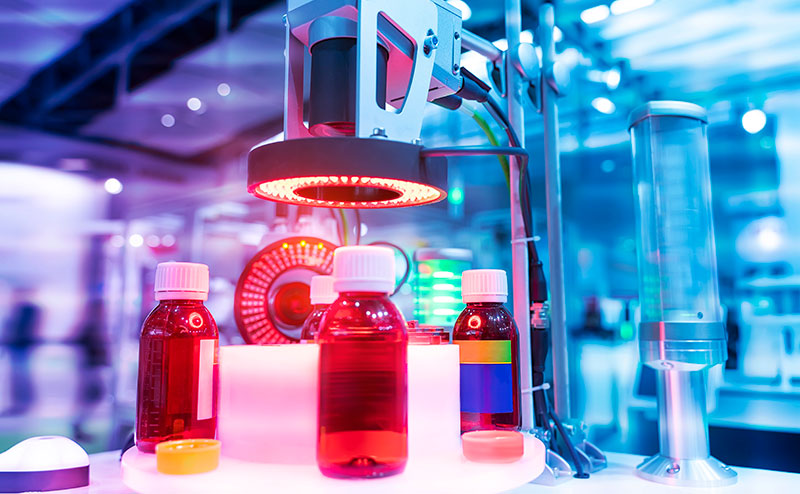
That’s because vision technology is not just for the big guys. As Peter Cavallo of Denso Products and Services Americas Inc. reinforces: “It is highly applicable to small and medium-sized businesses because vision today is reasonable in cost.”
Instead of a full-scale overhaul, manufacturers can gradually integrate ML and computer vision by targeting specific processes that will benefit the most.
This approach minimises risk and allows for a smoother transition, with middleware also being able to bridge the gap between AI systems and legacy machinery, enabling seamless communication and data exchange.
Although there are barriers to its adoption, the rewards of vision technology are clear. Increased productivity, smarter systems, and more efficient manufacturing processes await those who embrace this innovative technology.
Contact Foxmere today for a no-obligation quote on automation parts ranging from replacement or obsolete hardware to modern machine vision systems.